RESEARCH
The long-term goal of our laboratory is to understand the computational principles that underlie the way neural systems realize adaptive behavior, decision-making, and associated learning. In particular, we focus on (1) reward-based learning and decision-making and (2) social learning and decision-making. Toward this goal, we address computational questions by building computational and mathematical models. We also use human fMRI in combination with quantitative approaches such as model-based analysis and neural decoding techniques. We further develop quantitative techniques and methods for realizing innovative data analysis in neuroscience. In collaborative work with experimental investigators, we investigate the topics of our interest, using various types of experimental data.
THEMES
REWARD-BASED LEARNING AND DECISION-MAKING
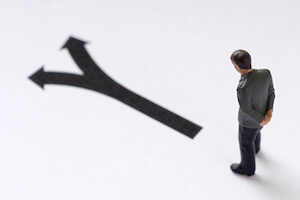
Decision-making is at the root of adaptive behavior. Decisions are often guided by reward prediction, and actions are often selected to maximize reward gain, using the prediction. Learning from experience is essential for being adaptive, to acquire appropriate prediction and selection in different environments. Decision-making is flexible and complex, and it can be best understood when behavioral-level complexity and related neural systems are mapped in computational frameworks that describe and model these systems. For instance, we use (and extend) a reinforcement learning framework to understand how reward prediction and action selection are learned and executed in relation to modeling and learning the environment. We examine the role played by neural mechanisms such as the frontal cortices, basal ganglia, and dopamine-reward circuits. We build computational and mathematical models and also conduct human fMRI studies, often in combination with modeling.
SOCIAL LEARNING AND DECISION-MAKING
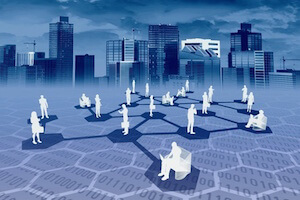
We aim to develop a quantitative theory of mind in decision-making by elucidating the neural mechanisms and neurocomputational primitives for how one understands the minds of others, predicts others’ behavior, and adjusts one’s behavior accordingly. We approach questions of social cognition by developing and using innovative quantitative frameworks such as reinforcement learning and Bayesian inference. For this purpose, we often combine human fMRI experiments with computational modeling. The results of this research can potentially be extended to computational psychiatry in the future, helping to identify differences in the prediction of others’ behavior between people with and without mental illness.
APPROACHES
Our main approaches are computational methods and human fMRI.
COMPUTATIONAL METHODS
Neural computation and modeling
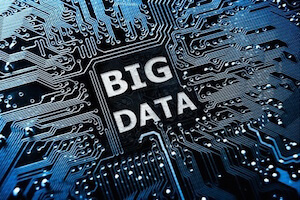
We use computational modeling and mathematical analysis in our research. Our aim is to find quantitative computational principles and to uncover the hidden properties of our topics of interest. In doing so, we identify processes that are often not directly evident in experimental findings, providing a normative understanding of the behavior and underlying processes, i.e., neural computations and circuits.
In consort with these works, we also develop effective learning and computation frameworks, such as reinforcement learning with representation learning and structural learning (e.g. Bayesian), their model-free and –based mechanisms, or generally brain-inspired AI; and we investigate how these computational machinery constitute of capabilities often psychologically termed such as motivation, emotion, planning, inference and pattern-symbolic interactions.
Neural coding and theoretical methodology
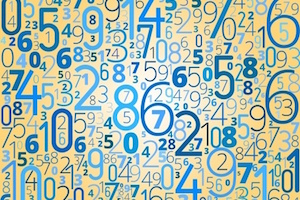
We develop novel methods and techniques for analyzing neural big data, and we devote particular attention to fMRI-related data. For example, we consider how to combine model-based analysis with decoding methods such as multivoxel pattern analysis (MVPA), or with resting-state fMRI and structural data analysis, in order to ask better questions and to find better ways to answer them. Method development must be closely connected with advanced theoretical techniques and requires familiarity with key experimental questions. Sometimes, we analyze neural data provided by our collaborators, including neurophysiological data from animal studies (e.g., dopamine neural activity, or massive calcium imaging data, from non-human primates and rodents).
HUMAN fMRI
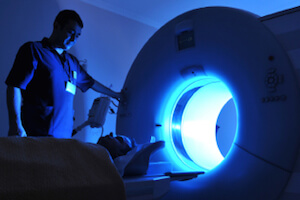
Human fMRI studies are performed in combination with computational modeling to study reward-based and social decision-making, and their associated learning. Computational models (e.g., reinforcement learning algorithms) allow us to specify a range of hypotheses and then test them. By fitting the models to the behavioral data and comparing different hypotheses, we can determine which model best accounts for a subject’s behavior. Using the processes and variables of the model, we can identify their neural correlates in the blood-oxygen-level-dependent (BOLD) signals of fMRI. This approach, called model-based analysis, allows us to identify key neurocomputational primitives. Compared with conventional approaches, this allows us to obtain a more precise mapping and deeper understanding of the computational processes underlying the neural activity involved in human decision-making. Model-based analysis also enhances computational studies through the building of models directly linked to behavioral and neural evidence. In addition, we are interested in combining model-based analysis with decoding techniques such as MVPA. Our human fMRI studies are conducted using our center's MRI systems with an excellent support from fMRI Support Unit and often in collaboration with excellent colleagues.
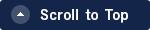